Home Solar Batteries: 2.2c Demand - Hourly & Smart Meter Models
​​
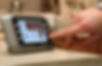
Introduction
This post specifically addresses the charging of domestic battery systems using solar PV and forms part of a series of entries looking to establish a reasonable view as to how the combination of solar PV and battery storage system should be modelled to ensure that information available to consumers should be considered accurate enough to establish a reasonable justification for investment in storage technologies.
If this is the first time you've accessed this information it is recommended that all of the relevant posts are considered in order through accessing the 'relevant posts' links
Hourly & Sub-Hourly Demand Models
In the previous posts in this series on demand data to be used in modelling battery storage in conjunction with solar PV systems we have considered data sources which would normally require the model to be based on period totals or be derived from those totals.
As discussed previously, any form of averaging solar PV generation effectively acts to smooth the level of variability and that it is the modelling of variability which creates a level of realism within the output of the required performance model, so when starting to delve into data below the day threshold we would need to not look at averages, but look to maintain variability by either employing actual long term energy consumption data, or to analyse long term usage and build a typical 'standard' usage pattern for the model to use.
Although Vriconian has used a bespoke setup to collect detailed consumption data for a considerable time, it is recognised that this would be a very rare position to be in, however, as the roll out of smart-metering continues the position is changing rapidly.
Smart-metering - Useful or Not?
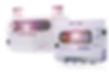
If configured to do so, smart-meters have the ability to meter & collect consumption data to a resolution of 30 minutes, this is generally referred to as HHM (Half Hourly Metering), which although not ideal (to be discussed later), certainly forms the most accurate data source available to the majority of households, providing the ability to crunch actual demand data through a battery storage performance model, however, the data available may not be of any use at all, depending on what is actually being measured.
For households where there are no existing micro-generation technologies, smart-meters will be measuring & recording household demand, which is the data format required for performance modelling.
Where solar PV or other grid connected microgeneration technologies already sit behind a smart-meter, collected data will reflect energy imports only, effectively rendering the data useless for demand modelling unless the export register is enabled and export data collected and made available on the same HHM basis as standard energy imports.
Demand Modelling
So far we've looked at data acquisition and analysis based on periods ranging from a basic annual total through to daily, however we'll now look into demand variability during the day using data collected on a hourly basis or via the HHM functionality as provided by a smart-meter and assess the effect of the size of the data collection buckets on period totals which would ultimately impact on the output of any battery performance model.
Let's expand on this and look to assess the impact of using hourly & HHM data buckets through example:
At Vriconian we have access to energy generation & consumption data collected to a 5minute bucket resolution, from which we are able to construct representations of hourly & half hourly data for direct comparison.
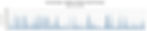
For the purpose of this exercise we'll select a weekend day with variable generation in the spring, in this case the Saturday nearest to mid-April and reconstruct both hourly & half hourly generation & demand datasets and compare the amount of energy energy each dataset would conclude as available to export against the actual 5 minute interval high resolution results.
Hourly Data Resolution
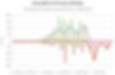
The chart above depicts 5 minute resolution data which has been consolidated into hourly data buckets, with the vertical bars representing hourly generation by a 4kWp solar PV system (green) & electricity consumption for the same period, both recorded as period Wh.
Overlaying the charted bars we show product of analysis of demand & supply to obtain the level of energy which is either available for diversion to battery storage (/export), or needs to be purchased (/imported) from the grid. In each case the red line represents the derived energy resulting from analysing hourly bucket data, with the blue & green lines showing the actual energy based on actual 5 minute data analysis.
As can be seen, during the day the hourly buckets are unable to capture the level of variation in demand & solar PV supply which, in terms of modelling, results in underestimation of energy required to be imported as well as what's available to export or divert to battery storage.
Half Hourly Data Resolution
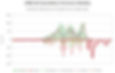
The same exercise performed on data consolidated into half hourly buckets so as to represent HHM data as collected by a smart-meter, the results charted using the same colour keys as above.
The change in data resolution clearly has an effect on the pattern depicted by the lines, yet the result stays the same in that modelling would still underestimate both daytime energy imports & exports, thus employing unnecessary inaccuracy even before we start to look at battery performance specifications & capabilities.
Sizing the discrepancy
So, we've looked at the pattern for hourly & half-hourly data buckets and visually compared variation to the underlying 5 minute data intervals, but in terms of totals within the day, how does this impact actual daily energy potentially available for batteries to store.
Constants
Solar PV Generation 12.6kWh
Energy Consumption 11.7kWh
Models
5 Minute HHM Hourly
Actuals
Export 7.6kWh 6.0kWh 5.4kWh
Import 6.7kWh 5.1kWh 4.5kWh
Summary
The analysis described above clearly shows the level of inaccuracy that the interrelationship between solar PV generation and household demand that can occur simply through applying data averaging on periods as short as just 30 minutes, this all resulting from smoothing out the natural variability which happens in the real world.
What needs to be understood is that the discrepancies detailed above aren't anything to do with different solar PV generation or household consumption, because they're using exactly the same data - it's just the timescale that the data applies to that's changing, in other words it's just the size of the bucket that's causing the difference.
A simple way to think about what we're seeing would be to leave one element of variability aside and consider solar PV generation to be 1.2kW, which resolves to 100Wh in each 5 minute period. Against this, for complete ease, we'll assume no base load at all and just one source of demand, boiling 2 litres of water in a 3kW kettle for around 5 minutes. Of course, consuming 3kW for 5 minutes equates to 250Wh (3000*5/60) of energy, however, if that energy is analysed over one hour the calculation would resolve to no import being necessary and 950Wh(1200-250) being available for export, whereas in the real world 3kW kettle is drawing 1.8kW (3-1.2) more power than the solar PV system is generating for 5 minutes, requiring 150Wh(1800*5/60) of daytime import.
It seems that hourly & even half hourly analysis smooth variability to a degree where considerable errors would be apparent in any battery performance model employing this level of detail, therefore output accuracy should be considered too low to have confidence enough to justify purchase decisions on potential energy savings.
This is part of a series looking at domestic Batteries
Please read in conjunction with other 'Related Posts' by using the links provided
Interested in what you've just read?
Have a look around the site & keep returning, it'll grow!