Home Solar Batteries: Performance Benchmarking - Pre-Analysed Datasets
- Opinion
- Mar 25, 2019
- 6 min read
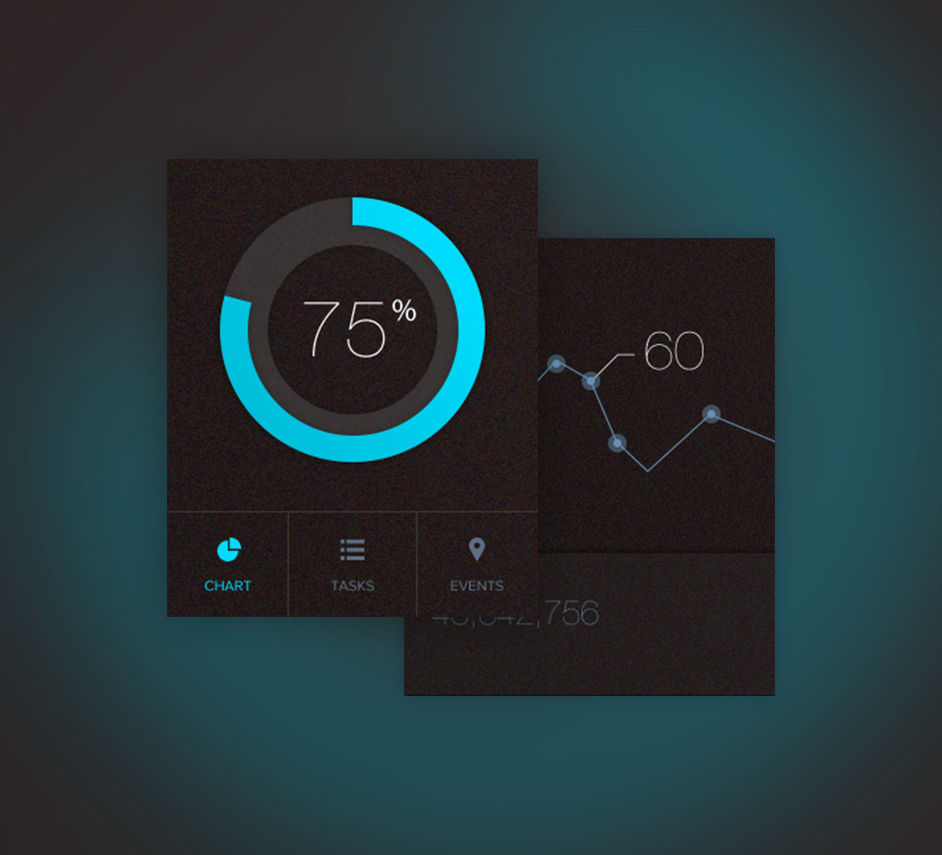
Introduction
This post specifically addresses the charging of domestic battery systems using solar PV and forms part of a series of entries looking to establish a reasonable view as to how the combination of solar PV and battery storage system should be modelled to ensure that information available to consumers should be considered accurate enough to establish a reasonable justification for investment in storage technologies.
If this is the first time you've accessed this information it is recommended that all of the relevant posts are considered in order through accessing the 'relevant posts' links
Simplifying the Dataset - An option when Benchmarking Performance ?

To date, this series has looked at the applying household energy consumption (demand) and solar PV generation (supply) on the calculation of excess solar PV energy which would be available to either export or divert to a battery storage solution.
In order to understand the level of detail which would be required to attain a reasonable level of accuracy we have considered various energy supply & demand data time-slices ranging from annual to sub-hourly and have attempted to explain how & why higher resolution data is needed in order to compare generation to household load on as close to a spot basis as possible in order to model the inherent variability and interrelationship of both supply & demand as opposed to applying averaged data, which tends to introduce smoothing mechanism inaccuracy into any model.
Earlier in the series we touched on the possibility of simplifying the battery storage modelling process through pre-analysing high resolution data and applying the results of this analysis to adjust & therefore improve the accuracy of longer period datasets, such as monthly household energy consumption & estimated solar PV system generation as calculated by tools such as PVGIS.
This is initially an interesting concept, which may be the route that many manufacturers have gone down when developing performance models for their own storage solutions. However, what needs to be considered is that for a manufacturer the battery performance is effectively a constant, with the variables being the differing generation & consumption patterns which apply to individual households and solar PV generation factors such as location, orientation and array capacity (kWp).
Although the models used by both manufacturers & installers may suit what they would like to convey about the performance of their own storage offerings, Vriconian expects that the majority interested in having battery storage solutions installed would effectively look to reverse the logic they employ and benchmark various solutions based solar PV supply & household demand being the constants and using the published battery specifications as the set of variables.
The concern with applying pre-analysed data to a simplified performance model lies in the methodology behind the analysis. For the analysis to provide the required level of accuracy to base sourcing justification on, it would need to be based on a study of a particular system's performance against both solar PV generation and household demand and would therefore only be applicable to systems displaying the same general performance attributes.
Two technically similar specification systems (in terms of rate of charge, discharge & storage capacity) would be expected to perform the same and deliver the same results, therefore laying the foundations for a pre-analysis simplified model. However, substantially change any one of the storage variables and the validity of the model changes, for example:-
Rate of Charge (kW)
Changing the rate at which the battery system accepts charge when solar PV generation is in excess to household demand can severely impact on the amount of energy being diverted to storage, resulting in a considerable difference in the overall performance of a combined solar PV microgeneration & battery storage solution, particularly when generation is highly variable (such as a mainly cloudy day with sunny spells) or when short-term high demands (such as a 3kW kettle) exist. In changing the interrelationship between the pattern of charge to that of discharge we effectively nullify the validity or accuracy of any simplified pre-analysed dataset.
We can see & gauge the effect of this through example :-
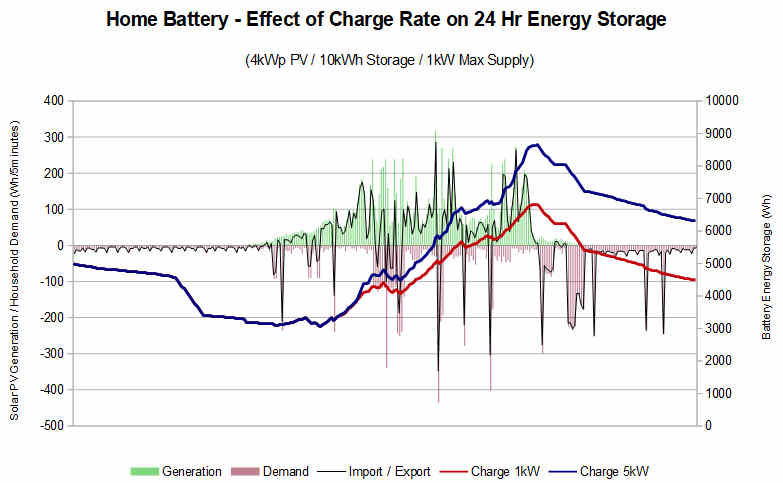
The chart above shows the effect of the rate at which a battery system's ability to receive charge has on the charge status. For this exercise a 4kWp solar PV system has been modelled against a 10kWh capacity battery storage solution with a power supply rating of 1kW with alternative maximum charge rates of 1kW & 5kW. The charge & supply control is assumed to be accurate and able to respond to condition changes within a matter of seconds and round trip efficiencies through the storage process are of many published storage solution specifications.
The household demand & solar PV generation are charted over 24hours of a typical spring day (Saturday in April) and represented by the vertical green and red bars, with the netted import & export shown as a grey line. For each of these data elements, the unit of measure is to be read against the left axis as energy (Wh) per 5 minute interval.
The red and blue bold lines describe the charge status (Wh) of the 10kWh nominal battery capacity storage solution, both assuming an initial 5kWh (50%) opening charge at midnight with the only difference between the two being the maximum rate of charge, in this case 1kW(red) vs 5kW(blue).
As can be seen, capping the rate of charge has a significant effect on the energy which can be diverted to storage. In the example above we can see that applying a 1kW maximum charge rate results in a closing balance reduction in storage of over 10% (to 4479Wh), whilst the ability to charge at 5kW raises the opening balance for the next day by 26% (to 6300Wh). This wide range of charge acceptance is currently apparent between various manufacturer battery storage, therefore the impact on overall performance is extremely relevant to benchmarking.
The divergence between the 1kW & 5kW charge acceptance variants only occurs at the point where the solar PV generated electricity passes the 1kW lower threshold. It is at this point that any model simplification pre-analysis conducted on one system begins to lose it's accuracy when applied to the other, in this case a 36.4% variance on the day against the opening 5kWh of initial battery charge.
Storage Capacity (kWh)
Similar to the example above, increasing the battery storage capacity increases the likelihood of carrying over energy storage from one day to another and will therefore influence the period system performance results upon which the simplified model pre-analysis is based, thus also rendering it ineffective.
The examples above show that any change in the battery specification which significantly impacts on performance would require a separate & specific pre-analysed simplified dataset to be built before a full performance analysis can be performed with any degree of confidence.
Summary
Again we see that modelling variability based on high resolution data is key to providing a high degree of confidence in the results the model provides, therefore when considering the creation of benchmarked performance for systems with differing specification & capacity characteristics it's looking like the crunching of vast amounts of data is needed as opposed to developing a multitude of solution specific simplified models.
Although a manufacturer or supplier may chose to employ a simplified dataset approach within a tool to provide performance estimates for the products they offer, it must be appreciated that the product range is likely to be relatively limited, resulting in an equally limited number of model specific performance datasets being needed for any one manufacturer.
In order to benchmark the performance of the combination of home battery storage and solar PV microgeneration across a full range of manufacturers with various model, specification and capacity offerings, it is essential to maintain consistency in the benchmark data being employed, especially the basis of applying generation (supply) and consumption (demand) to the charge & discharge algorithms.
It is therefore our conclusion that the simplified dataset approach would not provide a reasonable basis for wide ranging analysis for solution benchmarking.
This is part of a series looking at domestic Batteries
Please read in conjunction with other 'Related Posts' by using the links provided
Interested in what you've just read?
Have a look around the site & keep returning, it'll grow!
Comments